|
SQAB Tutorial: Creating Artificial Organisms Animated by a Selectionist Theory of Adaptive Behavior Dynamics |
Saturday, May 23, 2020 |
3:00 PM–3:50 PM |
Marriott Marquis, Level M2, Marquis Ballroom 6 |
Area: SCI; Domain: Theory |
BACB/PSY/QABA CE Offered. CE Instructor: Jack McDowell, Ph.D. |
Chair: Marcus Jackson Marr (Georgia Tech) |
Presenting Authors: : JACK MCDOWELL (Emory University) |
Abstract: The evolutionary theory of behavior dynamics (ETBD) is a complexity theory, which means that it is stated in the form of simple low level rules, the repeated operation of which generates high level outcomes that can be compared to data. The low level rules of the theory implement Darwinian processes of selection, reproduction, and mutation. This tutorial is an introduction to the ETBD, and will illustrate how the theory is used to animate artificial organisms that behave freely, and continuously, in any desired experimental environment. Extensive research has shown that the behavior of artificial organisms animated by the theory successfully reproduces the behavior of live organisms, in qualitative and quantitative detail, in a wide variety of experimental environments, including concurrent ratio schedules with equal and unequal ratios in the components, and concurrent interval schedules with and without punishment superimposed on one or both alternatives. An overview and summary of the research testing the ETBD will be provided. The material interpretation of the theory as an instance of supervenient realism will also be discussed. Finally, possible future directions will be considered with an eye toward identifying the most valuable path or paths for future development. |
Instruction Level: Basic |
Target Audience: Behavior analysts interested in the basic science; individuals interested in computational theories of behavior or machine learning; individuals interested in modeling clinically significant human behavior. |
Learning Objectives: At the conclusion of the presentation, participants will be able to: (1) create artificial organisms animated by the selectionist theory; (2) run artificial organisms in experimental environments; (3) summarize empirical support for the theory; (4) consider possible material interpretations of the theory; (5) consider fruitful paths for further development of the theory. |
|
JACK MCDOWELL (Emory University) |
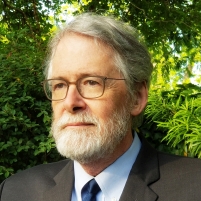 J. J McDowell received an A.B. from Yale University in 1972 and a Ph.D. from the State University of New York at Stony Brook in 1979. After completing his clinical internship, he joined the faculty of Emory University, where he is currently a professor in the Department of Psychology. Dr. McDowell is also a licensed clinical psychologist, and maintains a private practice of behavior therapy in Atlanta. Dr. McDowell's research has focused on the quantitative analysis of behavior. He has conducted tests of matching theory in experiments with humans, rats, and pigeons, has made formal mathematical contributions to the matching theory literature, and has proposed a computational theory of behavior dynamics. He has also written on the relevance of mathematical and computational accounts of behavior for the treatment of clinical problems. Dr. McDowell's current research is focused on his computational theory of selection by consequences, including studies of behavior generated by the theory's genetic algorithm, and possible implementations of the theory in neural circuitry. His work, including collaborations with students and former students, has been funded by NIMH, NSF, and NIDA. Dr. McDowell is a Fellow of the Association for Behavior Analysis International. |
|
|